Customer Story
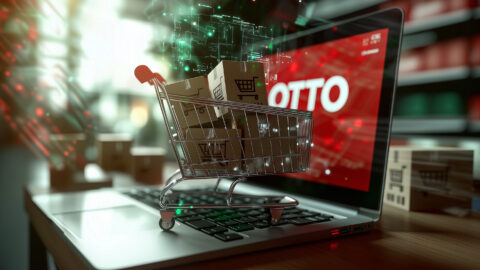
OTTO & Exasol Semantic Layer (ESL)
Reduced code complexity by 90%, enabling business users to access insights instantly with Exasol’s Semantic Layer—fully integrated into their infrastructure.
By leveraging advanced data infrastructure and machine learning, WashTec AG, the global leader in car wash solutions, enhanced its operations and customer experience. This initiative yielded significant benefits:
WashTec not only reinforced its market leadership but also established new benchmarks for efficiency and service quality in the car wash industry.
Germany
1800+
Car Wash
Exasol
The company is operating in over 80 countries with 1,800+ employees. They offer a range of products including gantry, tunnel, and self-service car washes, along with support services. With 50,000 installations washing 3 million vehicles daily, WashTec is a leader in the industry.
To support its digital transformation and enable more sophisticated data analysis, WashTec undertook a significant evolution of its data infrastructure. This overhaul was necessary to handle the increasing volume and complexity of data generated by their connected machines and digital services.
As shown in Figure 1, the company implemented Exasol as its central data warehouse, chosen for its high performance and scalability. This move allowed Washtec to consolidate and analyze large volumes of data from its connected machines. The company then integrated multiple data sources into this central repository, including SQL databases, NoSQL databases, and JSON documents from machine software. To streamline the data integration process, WashTec adopted Data Virtuality, using it to orchestrate data pipelines from these disparate sources into Exasol.
To address the critical challenge of unexpected machine failures, Washtec implemented a machine learning solution combining Exasol’s high-performance Analytics Engine with Dataiku’s ML platform. Led by data scientist Diana Scheidt, the team focused on delivering immediate business value through predictive maintenance while building a foundation for future ML innovation.
The integration between Exasol and Dataiku enables Washtec to process vast amounts of machine data in real-time while rapidly developing and deploying ML models.
Washtec’s first major machine learning initiative focused on predictive maintenance for gearboxes used in side brushes in their car wash machines. This project aimed to address a critical issue of unexpected failures that caused significant downtime, service disruptions, and potential revenue loss for customers. By developing a predictive maintenance solution, WashTec sought to enhance machine reliability and customer satisfaction.
From AI to DWH, we’ve got you covered.
Building on the success and lessons learned from their predictive maintenance model, Washtec is exploring additional machine learning use cases to further enhance their operations. The next project in their pipeline focused on wash count forecasting based on weather conditions. This initiative aimed to predict the number of car washes that would occur at a given location based on local weather forecasts and historical data.
By accurately forecasting wash volumes, Washtec sees potential for significant improvements in resource optimization and planning. This could help their customers better manage staffing levels, chemical inventories, and other operational resources, ultimately leading to improved efficiency and cost savings. The wash count forecasting project demonstrates Washtec’s commitment to leveraging data and ML across various aspects of their business, continuously seeking new ways to add value for their customers.
Through addressing these challenges, Washtec learned several valuable lessons:
By concentrating on concrete problems like gearbox maintenance prediction, Washtec was able to demonstrate tangible value quickly and build momentum for their AI initiatives.
By engaging front-line workers, especially service technicians, Washtec gathered crucial insights into real-world conditions and challenges. This feedback was instrumental in refining models and ensuring AI-driven insights were truly actionable and beneficial.
Washtec learned that deploying a model was just the beginning. Regular assessment of model performance, coupled with ongoing refinement based on new data and changing conditions, was essential to maintaining the effectiveness of their ML solutions over time.
By ensuring stakeholders understood how the models worked and what factors influenced their predictions, Washtec was able to build confidence in their AI-driven approach to maintenance and forecasting.
These best practices now inform Washtec’s approach to new ML projects, helping to ensure the long-term success and sustainability of their AI initiatives.
Building on the success of their digital transformation and initial ML projects, Washtec has plans for the future that align with their overall digital strategy:
The company is looking into leveraging their data infrastructure and ML capabilities to enhance sustainability efforts and improve operational efficiency across their car wash systems. This aligns with their commitment to innovation and environmental responsibility.
To support their growing machine learning workloads, Washtec continues to optimize their data infrastructure. Core to this is Exasol’s high-performance engine, which enables rapid model iteration and testing without the long processing delays typically associated with ML development. With data volumes growing from their expanding base of connected machines, the platform needs to maintain fast query performance while streamlining real-time data pipelines and expanding storage capacity for historical analysis
As new AI technologies continue to develop, Washtec aims to stay at the forefront of innovation, exploring potential applications of AI to further enhance their products and services. This could include areas such as computer vision for quality control or natural language processing for improved customer service.